Publications
Teratology Primer, 3rd Edition
Can Teratogenic Risk Be Predicted from Chemical Structure?
Grace Patlewicz, National Center for Computational Toxicology (NCCT), US EPA, 109 TW Alexander Dr, Research Triangle Park, NC 27711, USA
Disclaimer: The views expressed in this article are those of the authors and do not necessarily reflect the views or policies of the U.S. Environmental Protection Agency. Mention of trade names or commercial products does not constitute endorsement or recommendation for use.
Background concepts and definitions
The founding principles of in silico approaches are based on the premise that the properties of a chemical are inherent in its molecular structure. i.e. the (biological) activity of a chemical is a function of its molecular structure where activity can make reference to toxicity effects. This premise offers the possibility of developing models that predict the toxicity of a chemical based solely on its chemical structure. Such models have the potential to virtually screen large numbers of chemicals for their potential developmental toxicity as well as enable safety by design.
In practice, the way in which inferences of toxicity based on chemical structure are derived is by one of three main approaches: structure-activity relationships (SARs), quantitative structure activity relationships (QSARs) and chemical grouping approaches. A structure-activity relationship (SAR) is a qualitative association that relates a chemical (sub)structure (such as a functional group) to the presence or absence of a property or biological activity of interest. Often times SARs are referred to as structural alerts.
Figure 1. Examples of structural alert motifs
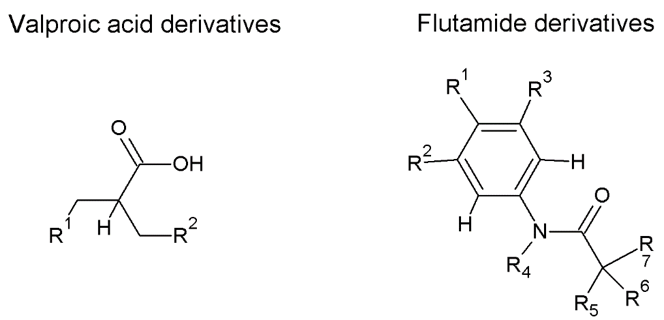
A QSAR, on the other hand, is a mathematical relationship (often a statistical correlation) relating one or more quantitative parameters derived from chemical structure to a property or biological activity of interest. These quantitative parameters are referenced as chemical descriptors and vary in terms of their complexity and computational needs. The simplest descriptors include those that account for the presence or absence of specific structural fragments or functional groups (fingerprints). Other descriptors encode whole chemical property information such as hydrophobicity, usually approximated by LogKow (the log of the octanol-water partition coefficient). There are descriptors that take into account 3D information and are based on quantum chemical calculations to characterize reactivity in parameters such as Energy of the Lowest Unoccupied Molecular Orbital (eLUMO) or Superdelocalizability, among others. QSAR models yield a continuous or categorical outcome.
Chemical grouping addresses the manner by which similar chemicals are grouped together typically based around some concept or aspect of chemical similarity in order for predictions to be made by a technique called “read-across”. In read-across, the activity/property information for one or more chemicals are used to predict the same property/activity for another chemical that is considered to be similar, usually on the basis of structural similarity.
Availability of existing (Q)SARs and expert systems for developmental and reproductive toxicity endpoints
While there are many (Q)SARs that have been published in the literature for a number of different biological activities, there is a paucity of (Q)SAR models in the literature for developmental toxicity. The reasons for this paucity are twofold: the lack of sufficient good quality data and a lack of knowledge about the mechanisms of action. We will discuss some of the potential opportunities herein.
The majority of the (Q)SARs that have been developed were derived on the basis of limited datasets focusing in on specific chemical classes such as short-chain carboxylic acids, substituted phenols, or haloacetic acids. There also have been (Q)SARs developed for the passive diffusion of chemicals across the placenta or other relevant barriers. A few of the published (Q)SARs have been based on larger heterogeneous datasets but their performance has typically been poor.
A number of these and other (Q)SAR models have been implemented into stand alone or web-based software applications for more convenient use. Such applications are known as expert systems and themselves can be categorized into one of three different types – statistical, knowledge-based, and hybrid. Statistical expert systems are based on a collection of QSAR models, knowledge-based systems typically rely on structural alerts, whereas a hybrid system is a combination of statistical and knowledge-based systems. A number of these systems are able to generate structure-based predictions of reproductive and developmental toxicity (DART) endpoints. Most of the (Q)SARs for DART toxicity are classification models making categorical predictions.
Examples of statistical expert systems include VEGA, Leadscope, TEST, TOPKAT, and CASE Ultra amongst others. VEGA (Virtual models for property Evaluation of chemicals within a Global Architecture) addresses a number of different human health-related endpoints, including a QSAR model that predicts whether a chemical might be associated with developmental toxicity. Leadscope Model Applier contains a suite of models for predicting developmental toxicity in the rodent fetus, including skeletal and visceral birth defects, fetal growth impairment, and fetal survival, and reproductive toxicity models for male and female rodents.
An example of a knowledge-based system is Derek Nexus v5.0.2 which contains over 850 structural alerts for a number of different toxicity endpoints. These alerts are supported by experimental toxicity data, a mechanistic hypothesis, example chemicals, and a reasoning engine to assign a level of confidence (certain, probable, plausible, etc.) for the endpoint prediction being made. Within the current version of Derek Nexus, there are approximately 60 alerts for reproductive toxicity, of which 50 alerts are specifically for teratogenicity. Other resources include OCHEM, a web-based resource which contains a collection of 12 ToxAlerts for developmental and mitochondrial toxicity.
An example of a hybrid expert system is TIMES (Tissue Metabolism Simulator) which contains a collection of structural alerts (or SARs), some of which are underpinned by QSARs based on 3D chemical information. A key feature of this tool is that it also contains a number of structure-metabolism relationships such that predictions can be made for chemicals taking into account their potential transformation products. TIMES does not have any specific models to predict developmental toxicity but it does contain models to predict estrogenic, androgenic, AHR binding activity, and aromatase inhibition.
Type of expert system |
Name |
Website |
Endpoint covered |
Knowledge based |
Derek Nexus |
Approx. 60 alerts covering teratogenicity, developmental toxicity and reproductive toxicity |
|
|
OCHEM |
12 ToxAlerts covering developmental and mitochondrial toxicity |
|
|
DART profiler |
Implemented in the OECD Toolbox |
Described in http://pubs.acs.org/doi/abs/10.1021/tx400226u |
Statistical |
VEGA |
https://www.vegahub.eu/ |
Binary classification class – developmental toxicant vs non-developmental toxicant |
|
Leadscope |
Developmental toxicity: Skeletal & visceral dysmorphogenesis, fetal growth restriction, fetal weight decrease, fetal survival (death, pre-implantation and post implantation loss) |
|
|
TEST (Toxicity Estimation Software Tool) |
https://www.epa.gov/chemical-research/toxicity-estimation-software-tool-test |
Binary classification class – developmental toxicant vs non-developmental toxicant |
|
MCASE/CASE Ultra |
Teratogenicity, fetal development and survival, reprotox (sperm toxicity and fertility), developmental toxicity and fetal dysmorphogenesis |
|
|
TOPKAT (TOxicity Prediction by Komputer Assisted Technology |
Binary classification class – developmental toxicant vs non-developmental toxicant |
|
Hybrid |
TIMES (Tissue Metabolism Simulator) |
http://oasis-lmc.org/products/models/human-health-endpoints.aspx |
Estrogen, Androgen and AHR binding affinity, Aromatase inhibition |
Table 1: Available expert systems and applications
Chemical grouping tools
There are also software tools to form chemical groups to enable read-across. Notable among these is the OECD QSAR Toolbox, a software application coordinated by the OECD (Organization for Economic Co-operation and Development), an intergovernmental organization. The Toolbox is designed to aid in the development, evaluation, justification, and documentation of grouping approaches to enable read-across predictions to be made. The system relies upon a workflow to help identify similar chemicals, form groups, and then perform predictions. A decision support system for DART effects was developed by researchers and comprised a set of structural alerts with associated mechanistic justifications. This decision tree is encoded in the OECD Toolbox enabling a more targeted search of similar chemicals that might share a common structural feature indicative of a common mechanism or mode of action. There are a number of other tools and resources to facilitate search of chemicals for read-across (see suggested reading).
An important consideration is being able to determine what data might be available for a given chemical or collection of chemicals in order to identify what the best approach might be to address specific data gaps and making toxicity predictions. There are many sources of available toxicity data that have been collected in different databases. Within the OECD Toolbox, data collections include the EU REACH data which comprises information submitted by companies to the European Chemicals Agency, and the Toxicity Reference database (ToxRefDB) compiled by EPA. The EPA Chemistry dashboard hosts links to different data and information sources and is linked back to chemicals and their associated chemical structures. These collections of data form a rich resource from which new models for developmental toxicity potentially could be derived.
Considerations for new model development and application
In recent years, advances in high-throughput technologies have offered new means to derive data helpful in elucidating the mechanistic pathways underpinning many of the endpoints of interest and moving away from reliance only on observations in animal studies. This has implications for the way in which future QSARs might be developed and used. Instead of QSARs linking structure to remote downstream toxicity effects in a simplistic correlative manner (such as is the case with some of the existing developmental toxicity QSARs), there could be scope to develop SARs and QSARs that capture a single step in a mechanistic pathway. Examples of such models include those already developed to predict in vitro estrogen binding affinity.
Validation of (Q)SARs has been a contentious issue for many years. Regulations in Europe provided momentum for reconsidering the manner and role that QSAR models could play in providing information for a range of different regulatory purposes. A set of 5 validation principles were formulated and agreed upon at an international level within the OECD. These were aimed to aid the development, application and interpretation of QSARs and their predictions for regulatory purposes. The 5 principles are: defined endpoint; unambiguous algorithm; a defined domain of applicability; appropriate measures of goodness of fit; robustness and predictivity; and, a mechanistic interpretation. These principles were intended to provide guidance for how a QSAR model and its prediction could be applicable for a given purpose. The principles aim to characterize the scientific validity of a model, rather than adhere to a formalized endorsement process of validation. Templates to assist in documenting QSAR models and their predictions made were also drafted to capture pertinent information, and these themselves were structured using the OECD principles as a foundation. There is still an open question of whether these principles and their associated documentation are sufficient to ensure greater uptake and acceptance of QSARs for regulatory purposes. There is certainly acceptance of using QSAR information as supporting information as part of an overall weight of evidence approach and, for certain endpoints, in lieu of experimental data. For developmental toxicity, positive predictions are considered helpful in such an overall assessment but since the available models typically provide a binary outcome (positive or negative outcome), their utility is limited. Of the principles, perhaps the greatest emphasis has been placed on the applicability domain of a model. This domain aims to describe the scope of the model in terms of where it can make reliable and robust predictions. The goal was to provide information to an end-user on when a prediction could be confidently relied upon.
Concluding remarks
For developmental toxicity effects, the preference has been to rely on read-across approaches to make inferences of toxicity. Unlike QSARs, read-across approaches have not had a formalized framework for assessing validity and robustness of the justification for the associated prediction being made for any specific purpose. However, this lack of a framework has been changing in the last few years with research efforts ongoing to develop ways and means of structuring, documenting justifications in a consistent manner and exploring to what extent high throughput screening (HTS) data might be useful in enhancing the confidence of a read-across prediction being made.
With the advent of data collections that have been made available – both conventional development toxicity data as well as HTS data sources, there are now more opportunities than ever before to exploit computational approaches to develop new predictive models for developmental toxicity endpoints.
Suggested Reading
U.S. EPA National Center for Computational Toxicology ‘s Chemistry dashboard https://comptox.epa.gov/dashboard provides resources relevant to computational toxicology.
QSAR validation principles and the OECD Toolbox can be found at: https://www.oecd.org/chemicalsafety/risk-assessment/oecdquantitativestructure-activityrelationshipsprojectqsars.htm
The JRC’s published review described many of the expert systems and literature (Q)SAR models available:
LoPiparo E, Worth AP. 2010. Review of QSAR Models and Software Tools for predicting Developmental and Reproductive Toxicity Elena Lo Piparo and Andrew Worth EUR 24522 EN Available at https://eurl-ecvam.jrc.ec.europa.eu/laboratories-research/predictive_toxicology/doc/EUR_24522_EN.pdf
The DART framework encoded in the OECD Toolbox is described in:
Wu S, Fisher J, Naciff J, Laufersweiler M, Lester C, Daston G, Blackburn K. 2013. Framework for identifying chemicals with structural features associated with the potential to act as developmental or reproductive toxicants. Chem Res Toxicol. 26(12): 1840-1861. doi: 10.1021/tx400226u.
Reviews of (Q)SARs validation, expert systems, read-across approaches and their integration are described in:
Patlewicz G, Fitzpatrick JM. 2016. Current and Future Perspectives on the Development, Evaluation, and Application of in Silico Approaches for Predicting Toxicity. Chem Res Toxicol. 29(4):438-51. doi: 10.1021/acs.chemrestox.5b00388.
Patlewicz G, Worth AP, Ball N. 2016. Validation of Computational Methods. Adv Exp Med Biol. 856:165-187.
Patlewicz G, Helman G, Pradeep P, Shah I. 2017. Navigating through the minefield of read-across tools: A review of in silico tools for grouping. Computational Toxicology 3: 1-18 https://doi.org/10.1016/j.comtox.2017.05.003
Worth AP, Patlewicz G. 2016. Integrated Approaches to Testing and Assessment. Adv Exp Med Biol. 856:317-342.